Young Faculty / 067
Assistant Professor Long Yin, Yoshida Laboratory, Department of Technology Management for Innovation
< Biography >
2015.4 - 2016.3 : National Institute for Environmental Studies, Social Systems Division, Associate Researcher
2019.9 : Department of Environment Systems, Graduate School of Frontier Sciences, The University of Tokyo, Ph.D.
2019.10 - 2020.4 : Tokyo University of Science, Assistant Professor
2020.5 - 2021.6 : The University of Tokyo, Institute for Future Initiative, Project Assistant Professor
2021.7 - present : The University of Tokyo, Graduate School of Engineering, Technology Management for Innovations, Assistant Professor
< About the research >
(A) My previous research, which was based on the Environmental-extended Input-output analysis, focused on the environmental impact embodied in a variety of economic activities, including the daily consumption behavior of residents. The ultimate goal of this research is to mitigate the negative anthropogenic impact on climate issues. Furthermore, studies were also carried out to assess the impact of human intervention on zero-emission and low-carbon social systems. EEIO analysis makes it possible to track the considerable quantity of indirect energy consumption in goods and services that are essential in daily life from production to consumption. Thus, the overall environmental consequences of all consumption behaviors can be regarded as our environmental "footprint" on the planet. For example, it is now possible to analyze carbon footprints derived from household daily consumption behaviors by income group, age group, and region (Fig. 1 shows the development of input-output analysis from a single region to multi-regional ones).
(B) The comparison and integration of traditional econometric models and data science are also accomplished through our previous investigations. For instance, we use the time series analysis of econometric and dynamic time warping (DTW) methods to examine the degree of similarity of time series data. Besides, a variety of machine learning methods, including K-nearest neighbor (KNN), decision tree, random forest, and XGBoost algorithm, are also involved in comparative research on the CO2 reduction potential of household lifestyles. Our current research focus is on how to apply data mining technology to real-world environmental research. In the era of big data, relevant research is also delivered to detect the collective consumption habits or lifestyles using features analysis methods in machine learning. Here, the most recent study is presented in the Figure below: by reclassifying the demographic features in the personal level consumption survey, it is possible to examine the prospect of promoting low-carbon behavior tailored to diverse consumption behaviors, particularly in the era of aging population. Furthermore, research is underway to quantify the environmental load involved in lifestyles by combining data on energy consumption in the macro-supply chain with micro-consumption data, with a focus on the demand side of the household sector. The purpose of this research is to increase the household sector's contribution to achieving carbon-neutral targets in the meantime of considering future trends such as declining birth rates and aging populations.
(C) Currently, the household sector accounts for the largest share of the final demand for goods and services across industries and is closely involved in all aspects of shaping a low-carbon society in the future. A change in lifestyle (behavioral change) is likely to lead to ripple effects on the consumption of energy. Among all consumption behaviors, dietary choices have a major impact on the global environment, and they are deeply connected to local socio-economics and culture. However, existing studies on sustainable dietary habits in households are significantly scattered in their themes and focus. In many cases, research is conducted while separating the knowledge of nutrition science, environmental science, and consumer behavior science. Hence, it is difficult to ensure the "consistency" in policy regarding a sustainable food environment. The current food production and consumption patterns are taking a toll on the environment and natural resource base. Meanwhile, unhealthy diets and malnutrition are among the top ten risk factors associated with disease burden. According to WHO, poor dietary habit is among the major contributors to the increasing incidence of all forms of malnutrition. Therefore, both environmental (global environmental health) and nutritional (individual level health) points of view should be considered to realize a sustainable and nutritionally balanced diet. This study aims to identify socio-culturally acceptable and economically accessible proposals for promoting a sustainable diet and improving the health and nutritional status of residents. Specifically, the research content can be summarized as follows: (1) Based on big data in food recipes and the technique of input-output analysis, we combine methods of conventional environmental economics and data science analysis to quantify the environmental load derived from individual dietary habits; (2) According to dietary habits by social attributes, the environmental load and nutritional intake condition are analyzed simultaneously through developing a new sustainable (environmentally and nutritionally) index (namely the Sustainable Dietary Index). In addition, recipe recommendations are provided in the light of residents' dietary preferences; (3) The potential of carbon reduction due to dietary habit changes is estimated, and scenarios based on these changes are formulated, taking into account Japan’s goal to achieve carbon neutrality in 2050 and SDGs 2, 3, 4, 6, and 7. In this way, the formation of sustainable dietary habits is examined from both theoretical and empirical perspectives. Through these efforts, it is expected that we will be able to propose sustainable dietary habits by demographics that account for both the nutritional aspect and the environmental load at the same time, with attention paid to the demographic structure.
< Future Aspirations >
In the future, I would like to make further contributions to research on achieving carbon neutral goals, especially regarding the household sector. This target is expected to be realized by combining multi-discipline knowledge, including environmental science, economics, ecology, nutritional science, and data science.
< URL >
Google scholar : https://scholar.google.com/citations?user=cUgJODwAAAAJ&hl=en
Researchmap : https://researchmap.jp/long
You May Also Like
These Related Stories
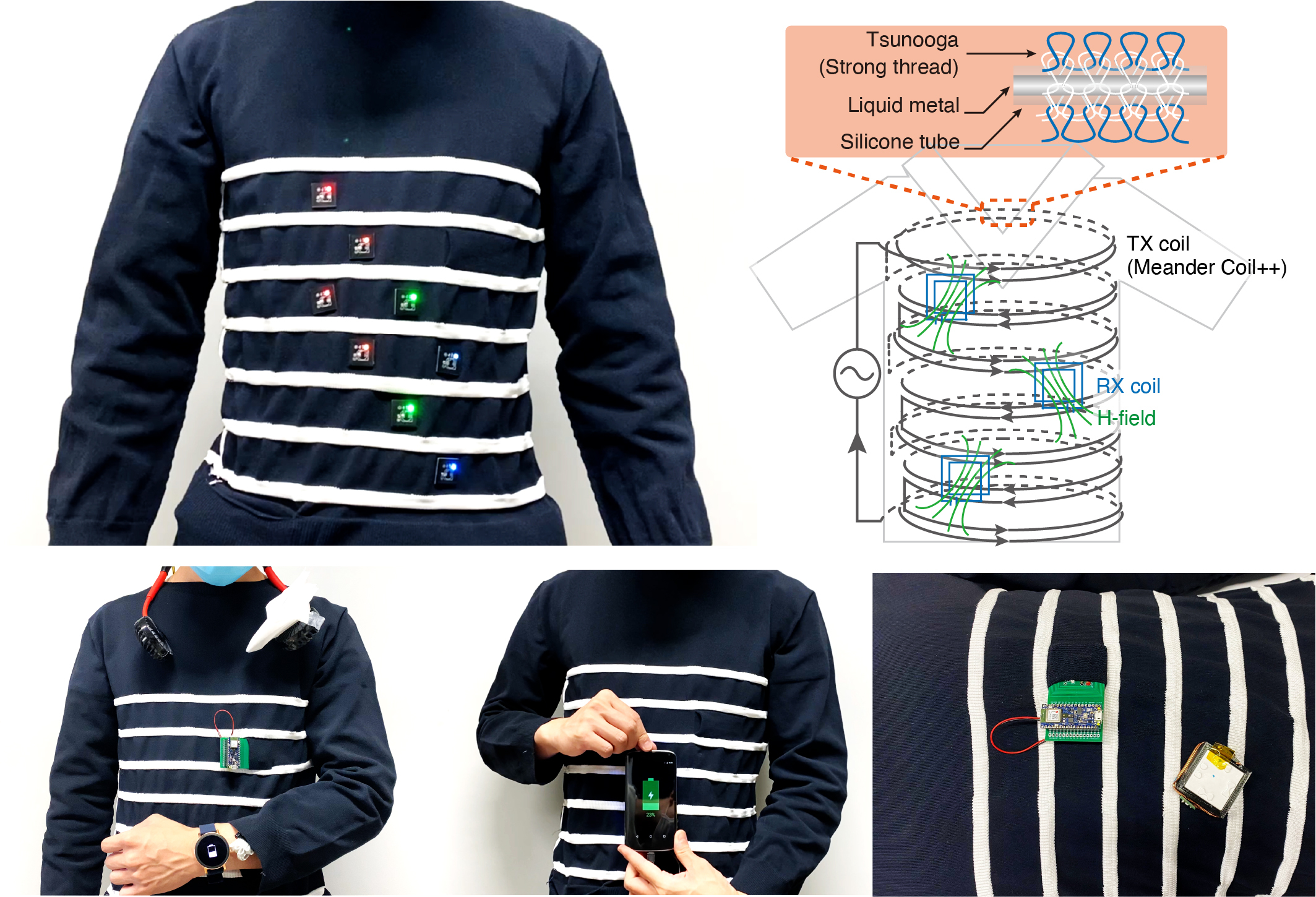
Ryo Takahashi (D3), Project Academic Specialist Wakako Yukita, Associate Professor Tomoyuki Yokota, Professor Takao Someya, and Professor Yoshihiro Kawahara received WISS 2022 Best Paper Award
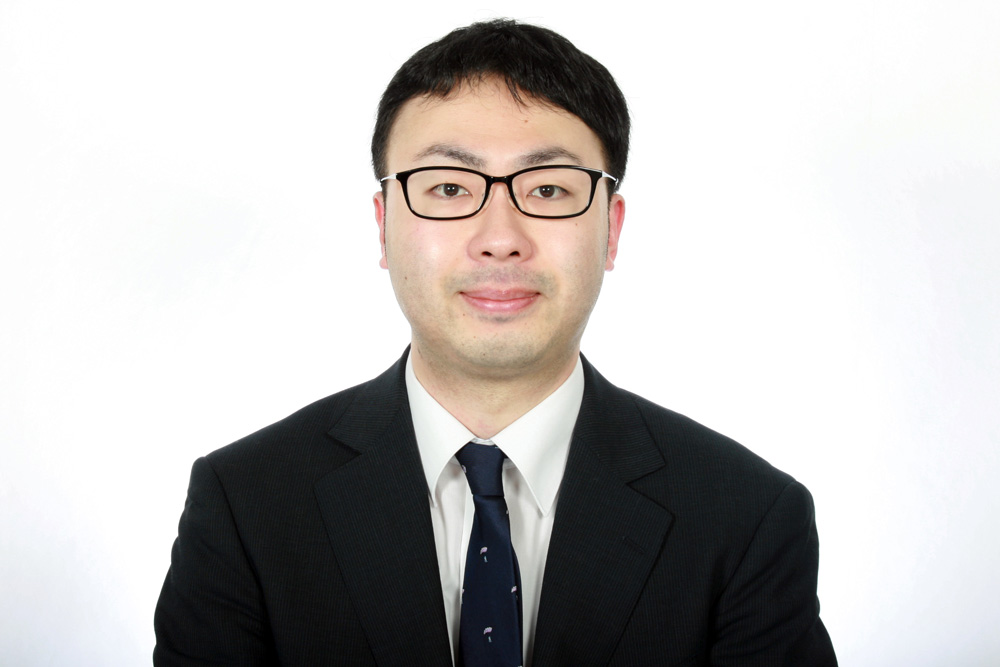
Young Faculty : Lecturer Takayuki Shiraiwa
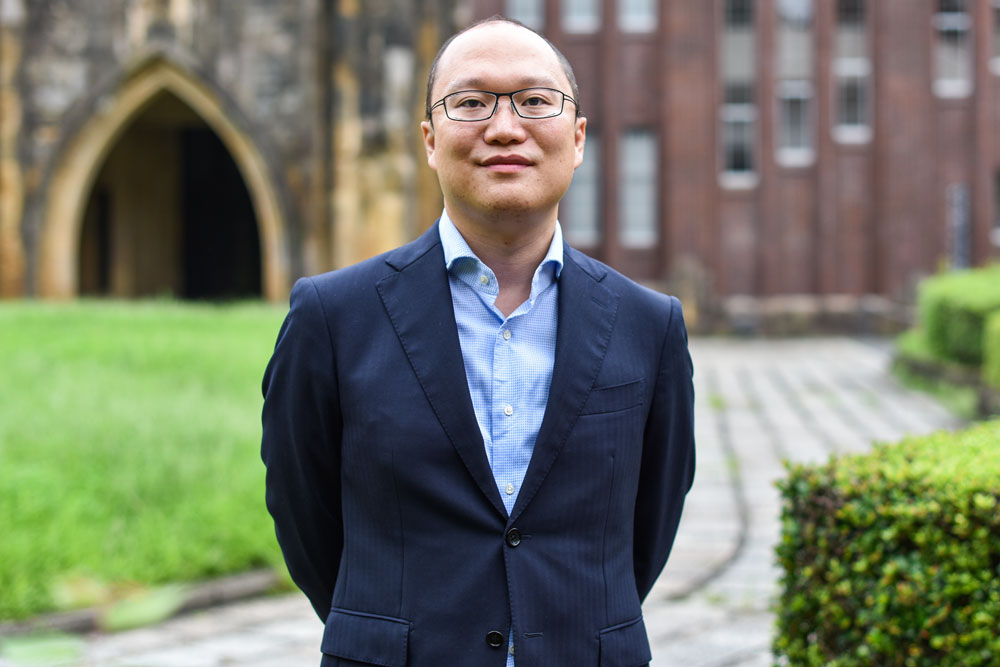